With Martin G. Moore
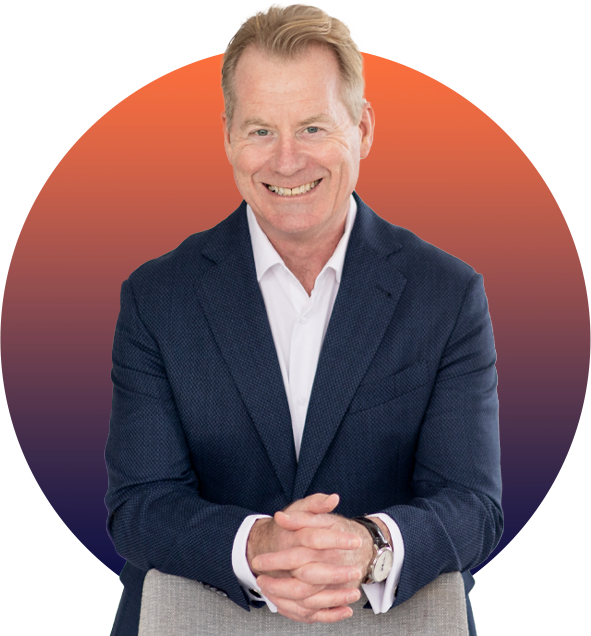
Was Lucy Letby wrongly convicted in her healthcare murder trial? We may never know… but what we do know is that her trial was plagued by serious flaws in the statistical evidence presented by the prosecution.
I find it just a little disturbing that the judge, lawyers, and jury were so innumerate, that they accepted the bogus statistics on face value.
This lack of numeracy is probably just as endemic in business! But everyone talks a good game, and it’s often difficult to see the glaring holes that lie just below the surface.
During my corporate career, I worked with a bunch of board directors, career politicians, and senior executives who exhibited a stunning lack of basic numeracy.
In today’s episode, I review the cautionary tale of the Lucy Letby trial; I take a look at how to build a robust process for decision-making; and I finish with eight ways to overcome the scourge of data illiteracy.
Generate Your Free
Personalized Leadership Development Podcast Playlist
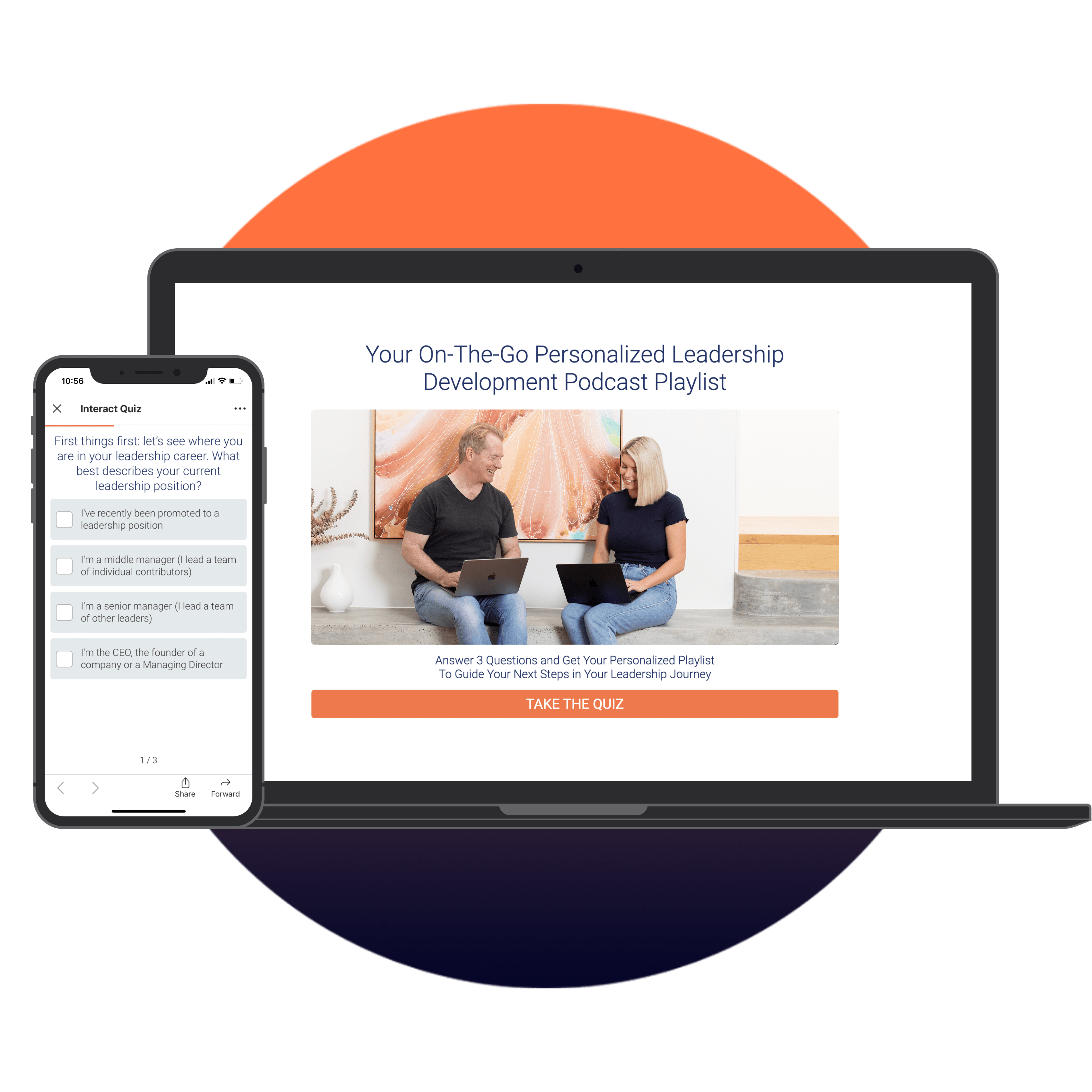
As a leader, it’s essential to constantly develop and improve your leadership skills to stay ahead of the game.
That’s why I’ve created a 3-question quiz that’ll give you a free personalized podcast playlist tailored to where you are right now in your leadership career!
Take the 30-second quiz now to get your on-the-go playlist 👇
Transcript
THE LACK OF DATA LITERACY IS STAGGERING
I came across an article in The Economist a few weeks ago and, to be perfectly honest, I found it just a little disturbing. It highlighted the complete lack of data literacy exhibited by key decision makers in the trial of Lucy Letby.
I shouldn’t have been overly surprised to learn that many people in key positions simply lack the education and the cognitive ability to prudently assess the information that’s presented to them.
As I read the article, I recognized a lot of the symptoms it described, and I was mildly triggered because I recalled just how much of this I saw during my corporate executive career. This happens even at the most senior levels, and no one is immune. I worked with a bunch of board directors, career politicians, and senior executives who exhibited a stunning lack of basic numeracy.
I begin today’s newsletter by reviewing the case of Lucy Letby. I then take a look at how to build a robust process for decision-making. And I finish with eight ways to overcome the scourge of data illiteracy.
LETBY’S TRIAL WAS STATISTICAL MALPRACTICE
I was absolutely fascinated by The Economist article, which was titled, The trial of Lucy Letby has shocked British Statisticians. It details the problems with the 2023 trial of a British nurse, who was convicted of multiple murders while working in the neonatal unit of a hospital in Chester, just south of Liverpool.
The article doesn’t protest Letby’s innocence, but rather, it points out the deeply problematic nature of her trial, which demonstrated an astonishing lack of understanding for the statistical evidence presented.
Letby was charged in 2020 with eight counts of murder and 10 counts of attempted murder. This was based predominantly on the unusually high number of infant deaths in the unit while Letby was on duty. In 2023, she was sentenced to life imprisonment without the possibility of parole.
This may or may not be a safe conviction – no one really knows. But what is abundantly clear is that a lot of the statistical data, which was critical in the jury reaching a guilty verdict was, at best, poorly understood and, at worst, dangerously misleading.
So poorly was this handled in fact that the Royal Statistical Society of Britain published a paper in response which was titled, Healthcare Serial Killer or Coincidence? These cases are quite different from a standard murder case.
In the vast majority of murder cases, the fact that a homicide has occurred isn’t in dispute, and from there it simply becomes a matter of working out if the person is actually guilty.
But in healthcare cases like Letby’s, which are often based on nothing more than circumstantial evidence, it’s sometimes not even clear that a homicide has taken place, let alone proving that a particular person is responsible for those deaths.
This is why it’s so important to understand things like probability, because the prosecutors are going to tell you that these events couldn’t possibly be a coincidence. When it comes to the fundamentals, it seems that the legal system and its protagonists are woefully ill-equipped to deal with them.
Statistics that are relied upon to prosecute individuals in healthcare cases like Letby’s exhibit a range of problems including:
A basic misunderstanding of what probability is;
Oversimplification of contributing factors; and
Investigative bias.
The Letby trial exposed the appalling lack of statistical literacy in the courts and judiciary. So, when you think about the basic competence of politicians, lawyers, judges, and other key professionals to understand the data they use to make decisions, I reckon you should be worried.
According to the Wikipedia entry on Letby’s case, one of the key pieces of evidence was a chart that showed Letby had been present for a number of deaths and other incidents in the neonatal unit. However, the chart omitted deaths and other incidents that had occurred when Letby was not present.
This is known as Texas sharpshooter fallacy, a phenomenon where differences in data are ignored, but similarities are overemphasized. It describes the tendency in human cognition to interpret patterns when none actually exist.
It’s like firing an arrow into a barn door and then painting a target around it so that the arrow is inside the bullseye.
One mathematics lecturer from the University of Oxford argued that, “You could make a chart like that for any nurse in any hospital”. He also said, “The spreadsheet duty roster is almost a textbook example, which I would give to my students of how not to collect and present data.”
DATA IS GENERALLY NOT WELL UNDERSTOOD…
The Economist article goes beyond the Letby trial to look at the broader implications that this low level of data illiteracy has on many of our key institutions.
It cited the example of Covid, where politicians making highly impactful decisions were unable to grasp the most fundamental concepts of statistics. Although it’s easy in hindsight to be critical of decisions that were made during Covid, it’s important to take some lessons from it.
Apparently, Boris Johnson, who was the prime minister of the UK at the time, was “bamboozled” by science and “struggled with the whole concept of doubling times”.
Without wanting to generalize, when you add the overlay of populist political bias and mainstream media pressure, it’s always going to be difficult to get prudent decisions from those in charge.
The Letby article explains how the principle of specialization contributes to this problem. British scientists are some of the most accomplished on the planet, but non-scientists are virtually illiterate when it comes to numbers.
Government data suggest that almost half of the working age population in Britain have the numeracy skills of a primary school child.
And statistics on the numbers of civil servants in the UK with STEM qualifications (Science, Technology, Engineering, and Mathematics), estimates put them at between 2% and 7%. In the US it’s almost 16%. And in South Korea, it’s around 30%. This isn’t particularly surprising, but it is no less worrying.
Think about the role that civil servants play:
They formulate fiscal policy;
They make recommendations on regulatory mechanisms; and
They advise politicians in areas where the minister has very little experience in their portfolio responsibilities…
… and their grasp of basic numeracy is questionable.
I was fortunate to be schooled in high level mathematics and physics, so it was relatively easy for me to analyze and interpret statistics. But then I started thinking about some of the decisions that were made above and around me during my corporate career.
I reflected on the hundreds of investment proposals that I analyzed where someone would put their case forward seeking a financial allocation, and the penny finally dropped for me: everyone pretends that they understand the numbers… and many people memorize the numbers so they can sound intelligent when they present them.
But it’s highly likely that many of the people I worked with in my corporate career simply didn’t have a grasp of the numbers that they were relying upon in their decision making.
HOW DOES DATA DRIVE YOUR DECISIONS?
I find this stuff super interesting – but that’s not much consolation for Lucy Letby is it!?
I want to bring this down to our own roles in our own companies. How do you implement a sound decision-making process in your team, while not losing sight of the likely deficit in the numeracy of the people involved?
For those of you who’ve read my book or studied Leadership Beyond the Theory, you’ll know that one of my seven pillars of leadership is, Make Great Decisions.
You never really know whether a decision was good, bad, or indifferent until you look in the rearview mirror. Hindsight brings wisdom. But you can predict whether a decision is likely to be good by looking at eight key elements:
Great decisions are made as close as possible to the action;
They’re made by a clearly accountable individual;
They’re made in consultation with the right experts (but, of course, not everyone);
They take into account both short-term and long-term implications;
They address the root cause of the problem, not just the symptoms;
They consider the holistic impacts of any potential decision;
Great decisions are communicated clearly; and, above all,
Great decisions are timely.
You’ll notice there is no mention of data or statistics here. That’s because data analysis weaves its way through a number of those criteria.
For example, the reason you make decisions at the lowest practicable level is that this is where people are most likely to have access to the data that enables the best decision to be made.
When you consult with other experts, you’re trying to glean data from broader sources, not just from your immediate perspective. Those experts should be prepared to present the facts, not just their opinions. As W. Edwards Deming once said, “In God we trust. Everyone else must bring data.”
The balance between short-term and long-term implications of any decision can only be properly determined with robust financial modeling and risk analysis.
If you can get the right data into the process, then it becomes a matter of understanding how much weight you should give to each element. This requires strong data literacy skills. If you don’t possess high level data literacy yourself, you’d better get someone close to you who does. Otherwise, you’re effectively flying blind.
The core leadership principle of excellence over perfection is key to analytical success. You can’t allow yourself to be overwhelmed by the data. You have to work out how much data is enough; how much analysis is enough; how much consultation is enough.
You reach the point of diminishing returns way faster than you think. But, if you aren’t data literate, you’re going to feel really insecure about not having sufficient data, and you’ll make a lot of bad decisions.
8 TIPS FOR IMPROVING YOUR DATA LITERACY
If you want to improve your ability to deal with the data inputs that any decision requires, you need to take a multi-faceted approach. You can’t just go out and hire a bunch of people with PhDs in statistical analysis. And, even if you could, that would really cause some other problems. Trust me.
Here are my eight top tips for improving your ability to draw accurate conclusions from any data that might be presented to you:
1. Test for numeracy when you hire. There’s a standard set of aptitude tests that can be easily applied when you hire anyone for any role. Verbal, numerical, and abstract reasoning can be easily and quickly assessed. Make sure that anyone you hire into a role that requires extensive exposure to numbers has high order numeracy skills.
2. Stop pretending. If you’re not confident in your ability to analyze complex data and understand statistical analysis, then be honest with yourself. Accept that this is the case. And instead of pretending you know what you’re doing, make sure you fill the gap. If you haven’t got it, go out and hire it.
3. Make sure you know the difference between correlation and causation. This is one of the most common mistakes that leaders make. You can find heaps of correlations in data sets, but they rarely represent a causal link. For example, there’s a correlation between drownings and ice cream sales – when one increases, so does the other. But no one would suggest that you are more likely to drown if you eat ice cream. It’s simply because both of these things tend to increase when the temperature is hotter.
4. Don’t be swayed by unsubstantiated opinions. Lots of people are going to tell you passionately and confidently why a certain course of action is necessary. You have to tune into their reasoning. If you do, you’ll often find things being presented as obvious facts when they’re nothing more than gut feel. Even your most persuasive communicators may have poor numeracy skills.
5. Uncover misleading biases. When you’re presented with any information, particularly when it’s part of a request for resources, you have to remember that the data has been framed to elicit a particular response. People cherry-pick data to support their case, so it requires some diligence to get underneath the assumptions. This is as much a function of leadership as it is data literacy. If you assume that the bias exists, you are much more likely to ask the type of questions that are going to uncover that bias and get you to a more level playing field.
6. Use your gut feel to test the numbers (not the other way around). We tend to start with our gut feel and experience, and then test the solution we think we want by applying some sort of supporting data. If we can learn to regulate this, we’re going to get much better results. Try to maintain a neutral position when you look at any data set. See if you can work out what that data is telling you. Once you feel as though you understand the analysis, only then should you ask the question, “Does that feel right?“
7. For big decisions, test a range of scenarios. Most often, we’re presented with a definitive answer to a problem, but it’d be so much more useful to have a range of different scenarios based upon different assumptions.
For example, a proposal might make an assumption on a currency exchange rate. It might assume that one AUD buys, say, 70c US. But what if that’s not how it pans out? What if the exchange rate increases to 75c US? What if it decreases to 65c US? How does that affect the economics of the proposal?
All assumptions should be tested, and a range of outcomes examined. Instead of saying, “This investment will earn a Net Present Value of $2.25 million,” it’s way more useful to say, “The most likely case is for a positive NPV of $2.25 million. However, if these assumptions don’t pan out, our worst-case scenario is a negative NPV of -$1.6 million, whereas our best possible case is a positive NPV of $3.9 million.”
8. Be inquisitive. Don’t assume anything. Ask a bunch of questions about any data that’s presented to you. Don’t just take it on face value. Ask questions like,
“Where did you source this data?”
“What assumptions have you made?”
“Have you tested the limits of those assumptions?
“How confident are you in your modeling?”
“If it all turns to custard, what is our maximum downside position?”
“Did you model any other options?”
And of course, my all-time favorite question,
“What’s your evidence for that?”
Asking smart questions is going to lead you to uncover the truth in the data.
BUILD THE CULTURE, BUILD THE CAPABILITY!
We started by looking at how a lack of data literacy can cause serious problems in critical situations. This is clearly egregious. Think of the thousands upon thousands of people who suffer the consequences of the low numeracy levels of our key decision makers.
In business, decisions that are based on bad assumptions are incredibly common. But you don’t have to fall into this trap. If you pay attention to what’s going on, and you’re attuned to the underlying bias in any numbers that you are presented with, it’s much more likely you’re going to make sound decisions.
Treat every unsupported assertion with the skepticism it deserves, and make sure your people know exactly what you expect. It’s as much about culture as it is about capability!
RESOURCES AND RELATED TOPICS:
Wikipedia entries:
Economist article:
The trial of Lucy Letby has shocked British statisticians
Royal Statistical Society paper:
Healthcare serial killer or coincidence?
Martin G Moore website: Here
The NO BULLSH!T LEADERSHIP BOOK – Here
Explore other podcast episodes – Here
Take our FREE 5 Day Leadership Challenge – Start Now
YOUR SUPPORT MATTERS
Here’s how you can make a difference:
Subscribe to the No Bullsh!t Leadership podcast
Leave us a review on Apple Podcasts
Repost this episode to your social media
Share your favourite episodes with your leadership network
Tag us in your next post and use the hashtag #nobsleadership